GBDT
GBDT分为ML Classification API和ML Regression API两大类模型接口。
模型接口类别 |
函数接口 |
---|---|
ML Classification API |
def fit(dataset: Dataset[_]): GBTClassificationModel |
def fit(dataset: Dataset[_], paramMap: ParamMap): GBTClassificationModel |
|
def fit(dataset: Dataset[_], paramMaps: Array[ParamMap]): Seq[GBTClassificationModel] |
|
def fit(dataset: Dataset[_], firstParamPair: ParamPair[_], otherParamPairs: ParamPair[_]*): GBTClassificationModel |
|
ML Regression API |
def fit(dataset: Dataset[_]): GBTRegressionModel |
def fit(dataset: Dataset[_], paramMap: ParamMap): GBTRegressionModel |
|
def fit(dataset: Dataset[_], paramMaps: Array[ParamMap]): Seq[GBTRegressionModel] |
|
def fit(dataset: Dataset[_], firstParamPair: ParamPair[_], otherParamPairs: ParamPair[_]*): GBTRegressionModel |
ML Classification API
- 功能描述
- 输入输出
- 包名:package org.apache.spark.ml.classification
- 类名:GBTClassifier
- 方法名:fit
- 输入:Dataset[_],训练样本数据,必须字段如下。
Param name
Type(s)
Default
Description
labelCol
Double
"label"
预测标签
featuresCol
Vector
"features"
特征标签
- 输入:paramMap、paramMaps、firstParamPair、otherParamPairs,fit接口的模型参数,说明如下。
Param name
Type(s)
Example
Description
paramMap
ParamMap
ParamMap(A.c -> b)
将b的值赋给模型A的参数c
paramMaps
Array[ParamMa p]
Array[ParamMa p](n)
形成n个
ParamMap模型参数列表
firstParamPair
ParamPair
ParamPair(A.c, b)
将b的值赋给模型A的参数c
otherParamPair s
ParamPair
ParamPair(A.e, f)
将f的值赋给模型
A的参数e
- 算法参数
算法参数
def setCheckpointInterval(value: Int): GBTClassifier.this.type def setFeatureSubsetStrategy(value: String): GBTClassifier.this.type def setFeaturesCol(value: String): GBTClassifier def setImpurity(value: String): GBTClassifier.this.type def setLabelCol(value: String): GBTClassifier def setLossType(value: String): GBTClassifier.this.type def setMaxBins(value: Int): GBTClassifier.this.type def setMaxDepth(value: Int): GBTClassifier.this.type def setMaxIter(value: Int): GBTClassifier.this.type def setMinInfoGain(value: Double): GBTClassifier.this.type def setMinInstancesPerNode(value: Int): GBTClassifier.this.type def setPredictionCol(value: String): GBTClassifier def setProbabilityCol(value: String): GBTClassifierdoUseAcc def setRawPredictionCol(value: String): GBTClassifier def setSeed(value: Long): GBTClassifier.this.type def setStepSize(value: Double): GBTClassifier.this.type def setSubsamplingRate(value: Double): GBTClassifier.this.type def setThresholds(value: Array[Double]): GBTClassifier
- 新增算法参数。
参数名称
参数含义
取值类型
doUseAcc
特征并行训练模式开关
True/False[Boolean]
参数及fit代码接口示例:
import org.apache.spark.ml.param.{ParamMap, ParamPair} val gbdt = new GBTClassifier() //定义def fit(dataset: Dataset[_], paramMap: ParamMap) 接口参数 val paramMap = ParamMap(gbdt.maxDepth -> maxDepth) .put(gbdt.maxIter, maxIter) // 定义def fit(dataset: Dataset[_], paramMaps: Array[ParamMap]): 接口参数 val paramMaps: Array[ParamMap] = new Array[ParamMap](2) for (i <- 0 to 2) { paramMaps(i) = ParamMap(gbdt.maxDepth -> maxDepth) .put(gbdt.maxIter, maxIter) }//对paramMaps进行赋值 // 定义def fit(dataset: Dataset[_], firstParamPair: ParamPair[_], otherParamPairs: ParamPair[_]*) 接口参数 val maxDepthParamPair = ParamPair(gbdt.maxDepth, maxDepth) val maxIterParamPair = ParamPair(gbdt.maxIter, maxIter) val maxBinsParamPair = ParamPair(gbdt.maxBins, maxBins) // 调用各个fit接口 model = gbdt.fit(trainingData) model = gbdt.fit(trainingData, paramMap) models = gbdt.fit(trainingData, paramMaps) model = gbdt.fit(trainingData, maxDepthParamPair, maxIterParamPair, maxBinsParamPair)
- 输出:GBTClassificationModel,GBDT分类模型,模型预测时的输出字段。
Param name
Type(s)
Default
Description
predictionCol
Double
"prediction"
Predicted label
- 使用样例
fit(dataset: Dataset[_]): GBTClassificationModel样例:
import org.apache.spark.ml.Pipeline import org.apache.spark.ml.classification.{GBTClassificationModel, GBTClassifier} import org.apache.spark.ml.evaluation.MulticlassClassificationEvaluator import org.apache.spark.ml.feature.{IndexToString, StringIndexer, VectorIndexer} // Load and parse the data file, converting it to a DataFrame. val data = spark.read.format("libsvm").load("data/mllib/sample_libsvm_data.txt") // Index labels, adding metadata to the label column. // Fit on whole dataset to include all labels in index. val labelIndexer = new StringIndexer() .setInputCol("label") .setOutputCol("indexedLabel") .fit(data) // Automatically identify categorical features, and index them. // Set maxCategories so features with > 4 distinct values are treated as continuous. val featureIndexer = new VectorIndexer() .setInputCol("features") .setOutputCol("indexedFeatures") .setMaxCategories(4) .fit(data) // Split the data into training and test sets (30% held out for testing). val Array(trainingData, testData) = data.randomSplit(Array(0.7, 0.3)) // Train a GBT model. val gbt = new GBTClassifier() .setLabelCol("indexedLabel") .setFeaturesCol("indexedFeatures") .setMaxIter(10) // Convert indexed labels back to original labels. val labelConverter = new IndexToString() .setInputCol("prediction") .setOutputCol("predictedLabel") .setLabels(labelIndexer.labels) // Chain indexers and GBT in a Pipeline. val pipeline = new Pipeline() .setStages(Array(labelIndexer, featureIndexer, gbt, labelConverter)) // Train model. This also runs the indexers. val model = pipeline.fit(trainingData) // Make predictions. val predictions = model.transform(testData) // Select (prediction, true label) and compute test error. val evaluator = new MulticlassClassificationEvaluator() .setLabelCol("indexedLabel") .setPredictionCol("prediction") .setMetricName("accuracy") val accuracy = evaluator.evaluate(predictions) println("Test Error = " + (1.0 - accuracy)) val gbtModel = model.stages(2).asInstanceOf[GBTClassificationModel] println("Learned classification GBT model:\n" + gbtModel.toDebugString)
- 结果样例
Test Error = 0.0714285714285714 Learned classification GBT model: GBTClassificationModel (uid=gbtc_72086dba9af5) with 10 trees Tree 0 (weight 1.0): If (feature 406 <= 9.5) Predict: 1.0 Else (feature 406 > 9.5) Predict: -1.0 Tree 1 (weight 0.1): If (feature 406 <= 9.5) If (feature 209 <= 241.5) If (feature 154 <= 55.0) Predict: 0.4768116880884702 Else (feature 154 > 55.0) Predict: 0.4768116880884703 Else (feature 209 > 241.5) Predict: 0.47681168808847035 Else (feature 406 > 9.5) If (feature 461 <= 143.5) Predict: -0.47681168808847024 Else (feature 461 > 143.5) Predict: -0.47681168808847035 Tree 2 (weight 0.1): If (feature 406 <= 9.5) If (feature 657 <= 116.5) If (feature 154 <= 9.5) Predict: 0.4381935810427206 Else (feature 154 > 9.5) Predict: 0.43819358104272066 Else (feature 657 > 116.5) Predict: 0.43819358104272066 Else (feature 406 > 9.5) If (feature 322 <= 16.0) Predict: -0.4381935810427206 Else (feature 322 > 16.0) Predict: -0.4381935810427206 Tree 3 (weight 0.1): If (feature 406 <= 9.5) If (feature 598 <= 166.5) If (feature 180 <= 3.0) Predict: 0.4051496802845983 Else (feature 180 > 3.0) Predict: 0.4051496802845984 Else (feature 598 > 166.5) Predict: 0.4051496802845983 Else (feature 406 > 9.5) Predict: -0.4051496802845983 Tree 4 (weight 0.1): If (feature 406 <= 9.5) If (feature 537 <= 47.5) If (feature 606 <= 7.0) Predict: 0.3765841318352991 Else (feature 606 > 7.0) Predict: 0.37658413183529926 Else (feature 537 > 47.5) Predict: 0.3765841318352994 Else (feature 406 > 9.5) If (feature 124 <= 35.5) If (feature 376 <= 1.0) If (feature 516 <= 26.5) If (feature 266 <= 50.5) Predict: -0.3765841318352991 Else (feature 266 > 50.5) Predict: -0.37658413183529915 Else (feature 516 > 26.5) Predict: -0.3765841318352992 Else (feature 376 > 1.0) Predict: -0.3765841318352994 Else (feature 124 > 35.5) Predict: -0.3765841318352994 Tree 5 (weight 0.1): If (feature 406 <= 9.5) If (feature 570 <= 3.5) Predict: 0.35166478958101005 Else (feature 570 > 3.5) Predict: 0.35166478958101 Else (feature 406 > 9.5) If (feature 266 <= 14.0) If (feature 267 <= 12.5) Predict: -0.35166478958101005 Else (feature 267 > 12.5) If (feature 267 <= 36.0) Predict: -0.35166478958101005 Else (feature 267 > 36.0) Predict: -0.3516647895810101 Else (feature 266 > 14.0) Predict: -0.35166478958101005 Tree 6 (weight 0.1): If (feature 406 <= 9.5) If (feature 207 <= 7.5) Predict: 0.32974984655529926 Else (feature 207 > 7.5) Predict: 0.3297498465552993 Else (feature 406 > 9.5) If (feature 490 <= 185.0) Predict: -0.32974984655529926 Else (feature 490 > 185.0) Predict: -0.3297498465552993 Tree 7 (weight 0.1): If (feature 406 <= 9.5) If (feature 568 <= 22.0) Predict: 0.3103372455197956 Else (feature 568 > 22.0) Predict: 0.31033724551979563 Else (feature 406 > 9.5) If (feature 379 <= 133.5) If (feature 237 <= 250.5) Predict: -0.3103372455197956 Else (feature 237 > 250.5) Predict: -0.3103372455197957 Else (feature 379 > 133.5) If (feature 433 <= 183.5) If (feature 516 <= 9.0) Predict: -0.3103372455197956 Else (feature 516 > 9.0) Predict: -0.3103372455197957 Else (feature 433 > 183.5) Predict: -0.3103372455197957 Tree 8 (weight 0.1): If (feature 406 <= 9.5) If (feature 184 <= 19.0) Predict: 0.2930291649125433 Else (feature 184 > 19.0) If (feature 155 <= 147.0) If (feature 180 <= 3.0) Predict: 0.2930291649125433 Else (feature 180 > 3.0) Predict: 0.2930291649125433 Else (feature 155 > 147.0) Predict: 0.2930291649125434 Else (feature 406 > 9.5) If (feature 379 <= 133.5) Predict: -0.2930291649125433 Else (feature 379 > 133.5) If (feature 433 <= 52.5) Predict: -0.2930291649125433 Else (feature 433 > 52.5) If (feature 462 <= 143.5) Predict: -0.2930291649125433 Else (feature 462 > 143.5) Predict: -0.2930291649125434 Tree 9 (weight 0.1): If (feature 406 <= 9.5) If (feature 183 <= 3.0) Predict: 0.27750666438358246 Else (feature 183 > 3.0) If (feature 183 <= 19.5) Predict: 0.27750666438358246 Else (feature 183 > 19.5) Predict: 0.2775066643835825 Else (feature 406 > 9.5) If (feature 239 <= 50.5) If (feature 435 <= 102.0) Predict: -0.27750666438358246 Else (feature 435 > 102.0) Predict: -0.2775066643835825 Else (feature 239 > 50.5) Predict: -0.27750666438358257
Regression API
- 功能描述
- 输入输出
- 包名:package org.apache.spark.ml.classification
- 类名:GBTRegressor
- 方法名:fit
- 输入:Dataset[_],训练样本数据,必须字段如下。
Param name
Type(s)
Default
Description
labelCol
Double
"label"
预测标签
featuresCol
Vector
"features"
特征标签
- 输入:paramMap、paramMaps、firstParamPair、otherParamPairs,fit接口的模型参数,说明如下。
Param name
Type(s)
Example
Description
paramMap
ParamMap
ParamMap(A.c -> b)
将b的值赋给模型A的参数c
paramMaps
Array[ParamMa p]
Array[ParamMa p](n)
形成n个ParamMap模型参数列表
firstParamPair
ParamPair
ParamPair(A.c, b)
将b的值赋给模型A的参数c
otherParamPair s
ParamPair
ParamPair(A.e, f)
将f的值赋给模型A的参数e
- 算法参数
算法参数
def setCheckpointInterval(value: Int): GBTRegressor.this.type
def setFeatureSubsetStrategy(value: String): GBTRegressor.this.type
def setFeaturesCol(value: String): GBTRegressor
def setImpurity(value: String): GBTRegressor.this.type
def setLabelCol(value: String): GBTRegressor
def setLossType(value: String): GBTRegressor.this.type
def setMaxBins(value: Int): GBTRegressor.this.type
def setMaxDepth(value: Int): GBTRegressor.this.type
def setMaxIter(value: Int): GBTRegressor.this.type
def setMinInfoGain(value: Double): GBTRegressor.this.type
def setMinInstancesPerNode(value: Int): GBTRegressor.this.type
def setPredictionCol(value: String): GBTRegressor
def setSeed(value: Long): GBTRegressor.this.type
def setStepSize(value: Double): GBTRegressor.this.type
def setSubsamplingRate(value: Double): GBTRegressor.this.type
参数及fit代码接口示例:
import org.apache.spark.ml.param.{ParamMap, ParamPair} val gbdt = new GBTRegressor() //定义回归模型 //定义def fit(dataset: Dataset[_], paramMap: ParamMap) 接口参数 val paramMap = ParamMap(gbdt.maxDepth -> maxDepth) .put(gbdt.maxIter, maxIter) // 定义def fit(dataset: Dataset[_], paramMaps: Array[ParamMap]): 接口参数 val paramMaps: Array[ParamMap] = new Array[ParamMap](2) for (i <- 0 to 2) { paramMaps(i) = ParamMap(gbdt.maxDepth -> maxDepth) .put(gbdt.maxIter, maxIter) } //对paramMaps进行赋值 // 定义def fit(dataset: Dataset[_], firstParamPair: ParamPair[_], otherParamPairs: ParamPair[_]*) 接口参数 val maxDepthParamPair = ParamPair(gbdt.maxDepth, maxDepth) val maxIterParamPair = ParamPair(gbdt.maxIter, maxIter) val maxBinsParamPair = ParamPair(gbdt.maxBins, maxBins) // 调用各个fit接口 model = gbdt.fit(trainingData) //返回GBTRegressionModel model = gbdt.fit(trainingData, paramMap) //返回GBTRegressionModel models = gbdt.fit(trainingData, paramMaps) //返回Seq[GBTRegressionModel] model = gbdt.fit(trainingData, maxDepthParamPair, maxIterParamPair, maxBinsParamPair) //返回GBTRegressionModel
- 输出:GBTRegressionModel或Seq[GBTRegressionModel],GBDT回归模型,模型预测时的输出字段如下。
Param name
Type(s)
Default
Description
predictionCol
Double
"prediction"
Predicted label
- 使用样例
fit(dataset: Dataset[_]): GBTRegressionModel样例:
import org.apache.spark.ml.Pipeline import org.apache.spark.ml.evaluation.RegressionEvaluator import org.apache.spark.ml.feature.VectorIndexer import org.apache.spark.ml.regression.{GBTRegressionModel, GBTRegressor} // Load and parse the data file, converting it to a DataFrame. val data = spark.read.format("libsvm").load("data/mllib/sample_libsvm_data.txt") // Automatically identify categorical features, and index them. // Set maxCategories so features with > 4 distinct values are treated as continuous. val featureIndexer = new VectorIndexer() .setInputCol("features") .setOutputCol("indexedFeatures") .setMaxCategories(4) .fit(data) // Split the data into training and test sets (30% held out for testing). val Array(trainingData, testData) = data.randomSplit(Array(0.7, 0.3)) // Train a GBT model. val gbt = new GBTRegressor() .setLabelCol("label") .setFeaturesCol("indexedFeatures") .setMaxIter(10) // Chain indexer and GBT in a Pipeline. val pipeline = new Pipeline() .setStages(Array(featureIndexer, gbt)) // Train model. This also runs the indexer. val model = pipeline.fit(trainingData) // Make predictions. val predictions = model.transform(testData) // Select example rows to display. predictions.select("prediction", "label", "features").show(5) // Select (prediction, true label) and compute test error. val evaluator = new RegressionE val gbtModel = model.stages(1).asInstanceOf[GBTRegressionModel] println("Learned regression GBT model:\n" + gbtModel.toDebugString)
- 结果样例
Root Mean Squared Error (RMSE) on test data = 0.0 Learned regression GBT model: GBTRegressionModel (uid=gbtr_842c8acff963) with 10 trees Tree 0 (weight 1.0): If (feature 434 <= 70.5) If (feature 99 in {0.0,3.0}) Predict: 0.0 Else (feature 99 not in {0.0,3.0}) Predict: 1.0 Else (feature 434 > 70.5) Predict: 1.0 Tree 1 (weight 0.1): Predict: 0.0 Tree 2 (weight 0.1): Predict: 0.0 Tree 3 (weight 0.1): Predict: 0.0 Tree 4 (weight 0.1): Predict: 0.0 Tree 5 (weight 0.1): Predict: 0.0 Tree 6 (weight 0.1): Predict: 0.0 Tree 7 (weight 0.1): Predict: 0.0 Tree 8 (weight 0.1): Predict: 0.0 Tree 9 (weight 0.1): Predict: 0.0
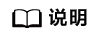
接口适用性说明:
- 本算法接口适用于基于鲲鹏服务器的HDP大数据平台,其中Java开发环境要求1.8及以上版本,Spark开发环境要求2.3.2版本, 低的HDP版本是3.1.0。
- 本算法运行在HDP大数据平台需部署的组件需要包括:HDFS、Spark2、Yarn、 ZooKeeper、Hive、MapReduce2。